Causation
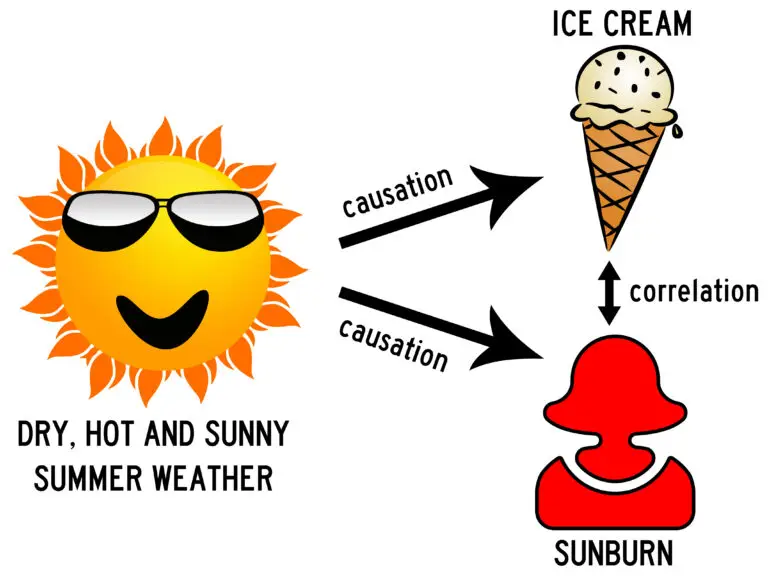
Table of Contents
What is Causation?
Causation in statistics refers to the relationship between variables where changes in one variable cause or influence changes in another variable.
Establishing causation is a fundamental aspect of statistical analysis, especially in experimental research, as it helps researchers understand the underlying mechanisms and effects of interventions or treatments.
Causal Inference
Causal inference is the process of determining whether changes in one variable directly cause changes in another variable. It involves identifying causal relationships based on empirical evidence and statistical analysis.
Criteria for Causation
- Temporal Order: The cause must precede the effect in time. In other words, changes in the independent variable(s) should occur before changes in the dependent variable(s).
- Association: There should be a consistent association between the cause and effect, meaning that changes in the cause are consistently accompanied by changes in the effect.
- Elimination of Alternative Explanations: Other potential explanations or confounding variables that could explain the observed relationship between the cause and effect should be ruled out or controlled for.
- Consistency: The cause-effect relationship should be observed consistently across different contexts, populations, and study designs.
- Dose-Response Relationship: In some cases, a dose-response relationship may strengthen the evidence for causation, where increasing levels of the cause lead to corresponding changes in the effect.
Types of Causation
- Direct Causation: Occurs when changes in the independent variable directly lead to changes in the dependent variable without the influence of other factors.
- Indirect Causation: Involves a chain of causation, where changes in the independent variable indirectly influence the dependent variable through intermediate variables or pathways.
- Spurious Causation: A misleading or false appearance of causation due to confounding variables or coincidental associations.
Experimental Research
In experimental research, causation can be established more confidently due to the ability to manipulate the independent variable(s) and control for confounding factors through random assignment and experimental design.
Randomized controlled trials (RCTs) are often used to assess causation by comparing outcomes between treatment and control groups.
Observational Studies
In observational studies, establishing causation is more challenging due to potential confounding variables and the inability to control for all factors that could influence the outcome.
Techniques such as regression analysis, propensity score matching, and instrumental variable analysis are used to strengthen causal inference in observational studies.
Counterfactual Framework
The counterfactual framework is commonly used in causal inference, where researchers compare observed outcomes with hypothetical outcomes that would have occurred in the absence of the cause.
Methods such as propensity score matching and difference-in-differences analysis rely on the counterfactual approach to estimate causal effects.
Causation Example
The Scenario
A researcher wants to investigate the relationship between studying hours and exam scores among high school students. The researcher hypothesizes that spending more time studying leads to higher exam scores.
The Process
Hypothesis: The researcher’s hypothesis is that studying hours (independent variable) cause changes in exam scores (dependent variable).
Study Design: The researcher collects data from a sample of high school students, recording their weekly studying hours and their exam scores.
Data Analysis: After collecting the data, the researcher performs statistical analysis to determine if there is a causal relationship between studying hours and exam scores.
Causation Analysis:
- Positive Causation: If the analysis shows that an increase in studying hours is consistently associated with higher exam scores, and this relationship is statistically significant after controlling for other factors, then the researcher may conclude that studying hours have a positive causal effect on exam scores.
- No Causation: If the analysis does not find a significant relationship between studying hours and exam scores, or if there are confounding variables that could explain the relationship (e.g., socioeconomic status, previous knowledge), then the researcher cannot establish causation. It may be that other factors are influencing exam scores, and studying hours alone may not be the primary cause.
Limitations and Cautions:
- Correlation vs. Causation: It’s essential to remember that correlation (a statistical relationship) does not imply causation (a cause-and-effect relationship). Additional research, experimental designs, or control for confounding variables may be needed to establish causation.
- Directionality: Causation implies that changes in one variable directly cause changes in another variable. However, in some cases, the relationship may be bidirectional or influenced by other factors.
Summary
In summary, establishing causation in statistics requires careful study design, data analysis, consideration of confounding variables, and cautious interpretation of results. While statistical analysis can provide insights into potential causal relationships, further research and evidence are often needed to confidently establish causation.
Related Links
Alternative Hypothesis
Association
Population
T-Test