Statistical Inference
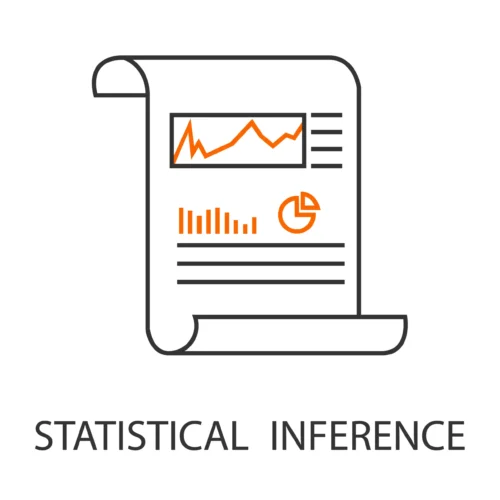
Table of Contents
What is Statistical Inference?
Statistical inference is a statistical process involving using sample data to make conclusions, predictions, or generalizations about a larger population. It allows researchers to draw inferences about population parameters based on sample statistics and provides a way to quantify uncertainty and variability in data.
Population and Sample
- A population refers to the entire set of individuals, items, or observations that interest a researcher.
- A sample is a subset of the population selected for data collection and analysis. Samples are used because studying the entire population is often impractical or impossible.
Parameter Estimation
- Statistical inference involves estimating population parameters (such as the mean, variance, proportion, etc.) based on sample statistics (such as the sample mean, sample variance, sample proportion, etc.).
- Point estimation provides a single value as an estimate of the population parameter, while interval estimation provides a range of values (confidence interval) within which the population parameter is likely to lie.
Hypothesis Testing
- Hypothesis testing is a key aspect of statistical inference that involves making decisions about population parameters based on sample data.
- The process typically involves setting up null and alternative hypotheses, selecting a significance level (alpha), calculating a test statistic, and comparing the test statistic to a critical value or p-value to determine whether to reject or fail to reject the null hypothesis.
Types of Hypothesis Tests
- Parametric Tests: These tests assume specific distributions for the data, such as the normal distribution, and include tests like t-tests, ANOVA, and z-tests.
- Nonparametric Tests: These tests do not make assumptions about the data distribution and include tests like the Mann-Whitney U test, Wilcoxon signed-rank test, and the Kruskal-Wallis test.
- One-Tailed and Two-Tailed Tests: In hypothesis testing, the alternative hypothesis can be one-tailed (specifying a direction of effect) or two-tailed (not specifying a direction).
Statistical Models
Statistical models, such as linear regression, logistic regression, and ANOVA, are used in statistical inference to describe relationships between variables, predict outcomes, and test hypotheses about those relationships.
Assumptions and Limitations
- Statistical inference relies on assumptions about the data and the methods used. Violations of these assumptions can affect the validity of the inferences.
- It’s important to consider the limitations of statistical inference, such as the need for representative samples, potential biases, and the impact of outliers.
Related Links
Analysis of Variance
Parameters
Population
Systematic Random Sampling